Asa Thibodeau, PhD
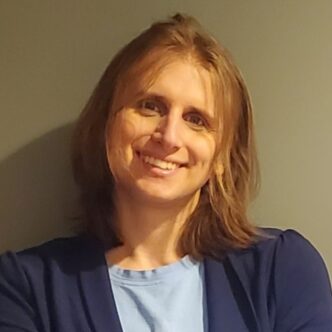
Machine Learning Models to Classify Regulatory Elements and Predict Their Gene Interactions from Clinical ATAC-seq Samples
Summary
Genome-wide association studies (GWAS) revealed that over 90% of disease-associated genetic variants are found in non-coding sequences that lead to dysregulated gene expression programs through disrupting regulatory elements (REs). REs are DNA sequences that mediate binding of proteins to DNA for regulating gene expression in a cell-specific manner and play a critical role in individual- and condition-specific gene regulation. An effective method for interrogating REs from clinical samples is Assay for Transposase Accessible Chromatin using sequencing (ATAC-seq), which captures genome-wide open chromatin regions (OCRs) with as little as 500-50,000 cells. By targeting OCRs, ATAC-seq effectively narrows the scope of where REs are found in a cell type of interest. However, determining a RE’s function (e.g., enhancer, insulator, etc.) and its target genes from ATAC-seq data alone remains a challenge. For this purpose, Classification of Regulatory Elements with ATAC-seq (CoRE-ATAC), was developed. CoRE-ATAC implements novel ATAC-seq data encoders that are used by a deep learning model to infer promoter, enhancer, and insulator classes of REs from OCRs. Training CoRE-ATAC on data from 4 cell types (Monocytes, GM12878, HSMM, and K562) achieved an average accuracy of 84% when applied on held-out test data from the same cell types. Moreover, high precision (~0.8) was observed after applying CoRE-ATAC on 40 samples across 7 cell types not used in model training, suggesting that CoRE-ATAC is an effective and robust model for determining RE function from ATAC-seq. Predictions from CoRE-ATAC will enable the development of future machine learning models for inferring enhancer-promoter interactions. Focusing on CTCF insulators, which have been shown to regulate chromatin structure by looping DNA, genomic regions that are most likely to be in close proximity and will be identified to infer target promoters/genes of predicted enhancers. These models will enable the study of epigenetic landscapes at the individual level, bringing us closer towards the development of individual specific therapies.
Being awarded the PhRMA Foundation Postdoctoral Fellowship in Informatics has enabled me to pursue research in areas that I’m the most passionate about as well as increased my confidence in designing research plans that have the potential to significantly impact future research and technologies. I am thankful for the PhRMA Foundation for supporting me as I transition into an independent researcher.